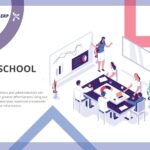
Using School Management Software to Track and Improve Attendance: A Comprehensive Approach
October 13, 2024In the age of digital transformation, the education sector has witnessed a paradigm shift, leveraging technology to optimize teaching methods, streamline administration, and enhance student outcomes. Among the technological advancements, machine learning (ML) stands out as a game-changer. By analyzing vast amounts of data, ML algorithms offer unparalleled insights, enabling schools to make data-driven decisions. This blog explores the role of machine learning algorithms in enhancing educational analytics and their potential to revolutionize the educational landscape.
Understanding Machine Learning and Educational Analytics
What is Machine Learning?
Machine learning is a subset of artificial intelligence (AI) that uses algorithms to identify patterns, learn from data, and make predictions or decisions without explicit programming. ML thrives on large datasets, and its applications are widespread, spanning healthcare, finance, retail, and now education.
Educational Analytics Defined
Educational analytics involves collecting, analyzing, and interpreting data generated within the education system to improve teaching, learning, and administrative processes. Data sources include student records, attendance, assessments, behavioral logs, and more. With ML algorithms, this data can be processed to uncover trends, predict outcomes, and recommend actions.
Applications of Machine Learning in Educational Analytics
1. Personalized Learning Pathways
Machine learning algorithms enable personalized learning by analyzing individual student data, including their strengths, weaknesses, learning styles, and pace. Key applications include:
- Adaptive Learning Platforms: ML-powered platforms assess students’ performance in real-time and adjust the difficulty of lessons or exercises accordingly. For instance, if a student struggles with algebra, the system can provide additional resources or simplified problems until they master the concept.
- Recommendation Systems: Just as streaming services recommend movies, ML algorithms suggest learning resources tailored to each student’s needs, such as videos, articles, or exercises.
2. Predictive Analytics for Academic Success
One of the most powerful uses of machine learning in education is predicting student outcomes. Algorithms analyze historical and real-time data to forecast:
- Grades and Performance: ML can predict how well a student is likely to perform in a course or subject based on past performance, attendance, and engagement levels.
- At-Risk Students: By identifying patterns such as declining grades or irregular attendance, ML helps schools pinpoint students at risk of dropping out or failing. Early identification allows for timely interventions, such as tutoring or counseling.
3. Enhancing Teacher Effectiveness
Machine learning can support teachers by providing insights into their instructional methods and identifying areas for improvement. Key features include:
- Classroom Analytics: ML algorithms can analyze classroom engagement data to identify teaching strategies that resonate most with students.
- Professional Development Recommendations: By assessing teaching patterns and outcomes, ML can suggest training programs or resources for teachers to enhance their skills.
4. Optimizing Administrative Processes
Beyond the classroom, machine learning enhances the efficiency of school administration through:
- Resource Allocation: ML algorithms analyze enrollment trends and resource usage to optimize class sizes, teacher assignments, and budgets.
- Predictive Maintenance: In facilities management, ML can predict when equipment or infrastructure needs maintenance, reducing downtime and costs.
5. Behavioral and Social Insights
Understanding student behavior is critical for creating a supportive learning environment. ML-powered analytics can:
- Detect Patterns: Algorithms identify behavioral trends, such as frequent tardiness or disengagement, enabling proactive measures.
- Sentiment Analysis: By analyzing student feedback, written assignments, or online discussions, ML can gauge emotional well-being and classroom morale.
6. Assessment and Grading
Machine learning transforms traditional assessment methods through automation and insights:
- Automated Grading: ML algorithms can grade assignments, quizzes, and even essays, saving time for teachers and providing consistent evaluations.
- Skill Gap Analysis: By analyzing test results, ML identifies specific areas where students struggle, allowing for targeted remediation.
Benefits of Machine Learning in Educational Analytics
1. Data-Driven Decision-Making
Machine learning transforms raw data into actionable insights, enabling schools to make informed decisions about curriculum design, teaching strategies, and resource allocation.
2. Improved Student Outcomes
With personalized learning and predictive analytics, ML empowers schools to cater to individual student needs, boosting academic performance and engagement.
3. Enhanced Efficiency
From automated grading to optimized resource allocation, ML streamlines administrative and academic tasks, freeing up time for educators to focus on teaching.
4. Scalability
Machine learning algorithms can handle large datasets, making them ideal for institutions of all sizes, from small schools to large educational networks.
Challenges in Implementing Machine Learning in Schools
While the benefits are immense, implementing machine learning in educational analytics comes with challenges:
1. Data Privacy and Security
Educational data often contains sensitive information. Ensuring data privacy and security is paramount when deploying ML solutions.
2. Equity in Access
Not all schools have the infrastructure or funding to implement advanced ML technologies, creating a digital divide.
3. Bias in Algorithms
ML algorithms can inadvertently perpetuate biases if trained on unrepresentative or flawed data. Ensuring fairness requires careful dataset curation and continuous monitoring.
4. Resistance to Change
Educators and administrators may be hesitant to adopt new technologies, especially if they are unfamiliar with ML’s potential or concerned about its impact on their roles.
5. Technical Expertise
Implementing ML requires skilled professionals to develop, deploy, and maintain algorithms, which may be a barrier for resource-constrained schools.
The Future of Machine Learning in Educational Analytics
The future holds immense potential for machine learning in education. Emerging trends include:
- Natural Language Processing (NLP): ML-powered tools that understand and analyze text will enhance student writing evaluation and provide personalized feedback.
- Virtual Learning Environments: Integration with virtual and augmented reality platforms will create immersive, ML-driven learning experiences.
- Real-Time Adaptive Systems: Advanced ML algorithms will continuously adapt instructional content based on real-time data, providing an even more personalized learning journey.
- Collaboration with IoT: IoT devices in classrooms will generate additional data, enabling more comprehensive analytics through ML algorithms.
- Global Insights: ML will facilitate collaboration across schools and districts worldwide, sharing best practices and insights for universal educational improvement.
Conclusion
Machine learning algorithms are revolutionizing educational analytics, transforming how schools track, analyze, and act on data. By enabling personalized learning, predictive analytics, and efficient administration, ML empowers educators to create more effective and inclusive learning environments.
However, to fully harness the potential of ML in education, schools must address challenges related to privacy, access, and expertise. With thoughtful implementation and ongoing innovation, machine learning will continue to play a pivotal role in shaping the future of education, ensuring that every student has the opportunity to reach their full potential.